# Enhancing AI Learning Through Brain Cell Diversity
Written on
Chapter 1: The Role of AI in Modern Life
Artificial Intelligence (AI) is becoming increasingly integrated into various facets of our lives, even if we are not yet facing scenarios akin to those in science fiction films like "Skynet." However, recent events, such as the Facebook outage, prompt us to reconsider the influence of AI on our daily routines.
While current AI does not constitute artificial general intelligence (AGI)—the type of sentient machine we often envision—the technology has made significant strides. Today's AI relies heavily on machine learning, showcasing remarkable capabilities across numerous fields. For instance, I've previously discussed its applications in areas such as scientific research, artistic creation, historical analysis, genetic advancements, mental health, aging studies (including the creation of "aging clocks"), video game ecosystems, Hollywood productions, astrobiology, epidemiology, stock markets, and employment trends.
Despite these advancements, AI systems still encounter limitations in their functionality. They excel in data processing and pattern recognition but are still evolving when it comes to deducing causality and learning from minimal datasets. Moreover, the ethical implications surrounding the creation and deployment of these technologies represent a critical area demanding further investigation.
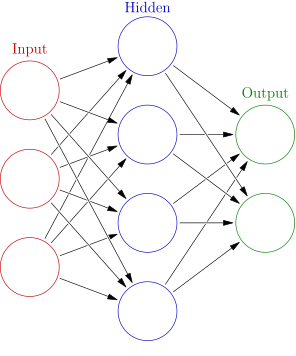
Chapter 2: Understanding Neural Networks
Leading AI technologies often utilize neural networks, which aim to replicate certain aspects of the human brain's structure: layers of interlinked neurons. In this system, the first layer receives inputs and transmits them through subsequent layers, each performing specific processing tasks until the information reaches the output layer. The complexity of these layers and the feedback mechanisms involved help classify different types of neural networks.
A crucial distinction between artificial and biological neural networks lies in the variation of their neurons. In artificial systems, neurons are uniform, whereas, in the human brain, each neuron is unique.
Section 2.1: The Importance of Neuronal Variation
A recent study has shed light on whether this variability in biological neurons offers any advantages or if it merely arises from the inherent complexities of biological systems. Researchers trained neural networks to identify visual and auditory signals while incorporating temporal heterogeneity among the neurons. This means that some artificial neurons took longer to process information than others.
The study yielded fascinating results:
- The introduction of temporal differences significantly enhanced performance. For the most complex auditory tasks, accuracy improved by approximately 15-20%, while simpler tasks showed no noticeable improvement.
- This diversity also bolstered the networks' generalization capabilities and resilience against erroneous learning.
The researchers concluded that neural diversity is an energy-efficient strategy for the brain. These heterogeneous networks perform comparably to homogeneous networks with many more neurons, but without the additional metabolic cost.
Interestingly, the temporal variability observed in the artificial neurons mirrored the patterns found in the brains of animals, including humans. This correlation suggests that our brains have evolved in a complex, temporally rich environment.
Neuroscience and AI: What artificial intelligence teaches us about the brain (and vice versa)
This video explores the relationship between neuroscience and artificial intelligence, examining how insights from one field can benefit the other.
Section 2.2: Broader Implications of Variation
While this study focused solely on temporal differences among neurons, it's essential to recognize that neuronal variability also encompasses structural and spatial dimensions. Variation is a critical element for enhancing AI systems.
Using Failures, Movement & Balance to Learn Faster
This video discusses how failures, movement, and balance contribute to accelerated learning in AI, emphasizing the importance of diverse experiences for optimal performance.
In summary, incorporating the natural variability found in brain cells into AI systems can significantly improve their learning processes and overall effectiveness. As research continues to unfold, the potential for more adaptive and efficient AI technologies appears promising.